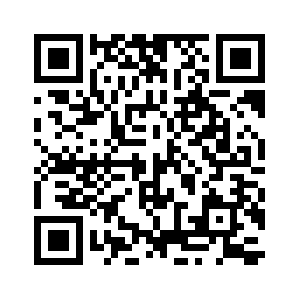
A Comprehensive Review of Federated Learning in Cancer Diagnosis and Prognosis Prediction
Artificial Intelligence收到 03 Mar 2025 接受 04 Apr 2025 在线发布 07 Apr 2025
Focusing on Biology, Medicine and Engineering ISSN: 2995-8067 | Quick Google Scholar
收到 03 Mar 2025 接受 04 Apr 2025 在线发布 07 Apr 2025
Federated learning (FL) has emerged as a promising approach for collaborative model training across multiple institutions without sharing sensitive patient data. In the context of cancer diagnosis and prognosis prediction, FL offers a potential solution to the challenges associated with data privacy and security. This paper reviews the application of FL in cancer diagnosis and prognosis prediction, highlighting its key benefits, limitations, and future research directions. We discuss the potential of FL to improve the accuracy and generalizability of predictive models by leveraging diverse and distributed datasets while preserving data privacy. Furthermore, we examine the technical and regulatory considerations associated with implementing FL in the healthcare domain. Finally, we identify opportunities for future research and development in FL for cancer diagnosis and prognosis prediction.
The current challenges in cancer diagnosis and prognosis prediction are indeed significant. The need for accurate, timely, and personalized assessments is crucial for improving patient outcomes [
]. Traditional methods, which often rely on small, localized datasets, can indeed limit the generalizability and predictive power of the models developed. This limitation can hinder the ability to accurately predict outcomes for a broader population of patients. Moreover, privacy concerns and data silos present additional obstacles. The sharing of patient information across institutions is essential for developing robust predictive models, but privacy concerns and data silos can impede this process [ ]. Finding ways to address these challenges while respecting patient privacy and data security is crucial for advancing cancer diagnosis and prognosis prediction.One potential solution to these challenges is the development of FL approaches. FL allows multiple institutions to collaborate on model training without sharing raw patient data. Instead, model updates are shared while keeping the data decentralized, thus addressing privacy concerns and data silos [
]. This approach has the potential to improve the generalizability and predictive power of cancer prognosis models by leveraging diverse datasets from multiple institutions. Additionally, advancements in technologies such as Artificial Intelligence (AI) and Machine Learning (ML) can help in developing more accurate and personalized predictive models. These technologies have the potential to analyze complex patterns in large datasets, leading to more precise predictions and personalized assessments for individual patients [ ].Furthermore, initiatives to promote data sharing and collaboration among institutions, while ensuring patient privacy and data security, are essential for overcoming the challenges associated with limited datasets and data silos. Establishing standardized protocols and frameworks for data sharing and collaboration can facilitate the development of more robust predictive models for cancer diagnosis and prognosis. Finally, addressing the challenges in cancer diagnosis and prognosis prediction requires a multi-faceted approach that leverages advanced technologies, promotes data sharing and collaboration, and prioritizes patient privacy and data security. By overcoming these challenges, we can work towards more accurate, timely, and personalized assessments that ultimately improve patient outcomes in the fight against cancer.
FL provides to address the challenges associated with sharing sensitive data in the healthcare industry. By allowing multiple institutions to collaborate on building a shared ML model without sharing raw data, FL helps preserve patient privacy while still enabling the creation of accurate predictive models [
]. In this approach, each institution retains control over its local data and trains the ML model on that data. Only the model updates or aggregated information is shared with a central server, which then combines these updates to improve the overall model. This way, sensitive patient data remains within the institution, reducing the risk of privacy breaches [ ].Furthermore, FL can lead to the creation of more comprehensive and accurate predictive models by leveraging diverse datasets from multiple institutions. This can result in better generalization and improved performance of the ML model, ultimately benefiting patient care and medical research. However, it's important to consider the technical and regulatory challenges associated with implementing FL in healthcare, such as ensuring the security of model updates during transmission and addressing regulatory requirements for data sharing across institutions [
, ]. Despite these challenges, the potential benefits of FL make it a compelling solution for collaborative ML in healthcare.FL is indeed a promising approach for improving the accuracy of cancer prognosis prediction while respecting patient privacy [
]. In this scenario, each hospital can utilize its own patient data to train a ML model. By keeping the data within each hospital's local environment, patient privacy is maintained, as the sensitive information doesn't need to be shared across institutions. The models' updates, rather than the raw data, are then aggregated to create a more comprehensive and accurate global model. This approach allows for the incorporation of diverse characteristics of cancer across different populations, as each hospital's model reflects the unique patient demographics and disease profiles specific to its location.Furthermore, FL can help mitigate issues related to data silos and data privacy regulations, as it enable collaborative model training without the need to centralize sensitive patient information [
, ]. This approach not only benefits the accuracy of the predictive model but also aligns with ethical considerations regarding patient privacy and data security. Overall, FL presents a promising avenue for advancing cancer prognosis prediction while upholding patient privacy and data security.FL indeed offers significant potential in the healthcare sector, particularly in the context of cancer prognosis prediction and personalized treatment planning [
]. By allowing hospitals to collaboratively train a shared model without sharing patient data, FL enables the aggregation of insights from diverse sources while maintaining data privacy and security [ ]. This approach is especially valuable in the field of oncology, where the heterogeneity of cancer types and patient populations necessitates a comprehensive and diverse dataset for accurate predictive modeling. By continuously updating the global model with new insights from individual hospitals, FL can capture the nuances of different patient demographics, disease presentations, and treatment responses, leading to more robust and generalizable predictive capabilities.Moreover, the ability to develop personalized treatment plans based on a broader and more representative dataset holds great promise for improving patient outcomes. By leveraging the collective knowledge embedded in the federated model, healthcare providers can tailor interventions to individual patients with greater precision, accounting for factors such as genetic variations, treatment histories, and comorbidities [
]. However, it's important to address challenges such as model drift, data distribution shifts, and maintaining model fairness and transparency in FL settings. Additionally, ensuring regulatory compliance and ethical considerations, such as informed consent and data ownership, are crucial aspects that need to be carefully navigated in the implementation of FL in healthcare [ ]. Overall, the collaborative and adaptive nature of FL presents a compelling opportunity to advance cancer care by harnessing the collective intelligence of healthcare institutions while safeguarding patient privacy and promoting data-driven, personalized medicine.FL indeed holds great promise for addressing the challenges in cancer diagnosis and prognosis prediction [
]. By allowing multiple institutions to collaboratively train a shared ML model while keeping patient data decentralized, FL enables the creation of more accurate and generalizable predictive models. This approach respects patient privacy by keeping sensitive data local and fosters collaboration among healthcare institutions, which is crucial for advancing cancer research and improving patient outcomes.One of the key advantages of FL is its ability to leverage diverse and distributed datasets from different healthcare institutions without the need to centralize patient data [
, ]. This is particularly important in the context of cancer research, as it allows for the development of predictive models that are more robust and representative of diverse patient populations [ , ]. By aggregating knowledge from multiple sources, FL can help overcome the limitations of single-site studies and improve the generalizability of predictive models. Moreover, FL addresses privacy concerns by keeping patient data within the boundaries of individual institutions. This decentralized approach minimizes the risk of data breaches and unauthorized access, thereby safeguarding sensitive patient information. As a result, healthcare institutions can participate in collaborative model training without compromising patient privacy, which is essential for building trust and fostering cooperation in the healthcare community.Furthermore, FL promotes collaboration among healthcare institutions by enabling them to jointly improve predictive models without sharing raw data. This collaborative approach encourages knowledge sharing and accelerates the development of more effective diagnostic and prognostic tools for cancer [
]. By pooling expertise and resources, institutions can work together to address common challenges and drive innovation in cancer research and clinical practice. Finally, FL offers a promising solution to the challenges in cancer diagnosis and prognosis prediction by facilitating the development of more accurate and generalizable predictive models while respecting patient privacy and fostering collaboration among healthcare institutions. As this approach continues to evolve, it has the potential to significantly impact the field of oncology by enabling the creation of advanced predictive models that benefit patients, clinicians, and researchers alike [ , ]. Table 1 presents a structured comparison of key Federated Learning (FL) studies in oncology.To provide a structured and systematic review, we adopt a mapping review approach. This method helps categorize the existing literature, identify gaps, and suggest future research directions. Literature from leading medical and AI research databases are considered and studies are categorized based on FL frameworks, cancer types, performance metrics, and regulatory considerations.
Biopsy: Biopsy is a crucial medical procedure used to diagnose various conditions, including cancer. There are different types of biopsies, such as needle biopsy, surgical biopsy, and endoscopic biopsy, each suited for different situations.
A needle biopsy is a minimally invasive procedure commonly used to obtain tissue samples for diagnostic purposes, as shown in Figure 1. In the case of a suspicious breast lump, a needle biopsy can provide crucial information about the nature of the lump, whether it is benign or malignant [
]. During the procedure, the patient is usually positioned lying down or seated, and the skin over the biopsy site is cleaned and numbed with a local anaesthetic to minimize discomfort. The physician then uses imaging techniques such as ultrasound, mammography, or MRI to guide the needle to the precise location of the abnormal tissue. Once the needle is in place, a small sample of tissue is extracted and collected for analysis.After the biopsy, the tissue sample is sent to a pathology laboratory where a pathologist examines it under a microscope. The pathologist looks for any abnormal cells or signs of cancer, such as changes in cell size, shape, and organization. The results of the biopsy help the healthcare team determine the appropriate course of treatment for the patient. In real-time, this procedure allows for a timely and accurate diagnosis, enabling healthcare providers to develop personalized treatment plans tailored to the individual patient's needs. It also minimizes the need for more invasive surgical procedures to obtain tissue samples, reducing patient discomfort and recovery time [
, ]. Overall, needle biopsies play a crucial role in the early detection and diagnosis of various medical conditions, including cancer, and contribute to improved patient outcomes.Picture a patient presenting with a concerning lump in their breast. In this case, a surgical biopsy might be recommended to obtain a larger tissue sample for a more comprehensive analysis [
]. The surgeon would remove the entire lump or a significant portion of it to ensure that the pathologist has enough tissue to make an accurate diagnosis. Another example could be a suspected tumor in the lungs. If a small needle biopsy, such as a fine needle aspiration, doesn't yield enough tissue for a conclusive diagnosis, a surgical biopsy, such as a thoracoscopic biopsy, might be performed to obtain a more extensive tissue sample. In both scenarios, the surgical biopsy allows for a more thorough examination of the tissue, which can be crucial for determining the nature of the abnormality and guiding treatment decisions. This type of biopsy is often necessary when a deeper or larger tissue sample is required to make an accurate diagnosis, especially in cases where the suspected abnormality is located in a hard-to-reach or deeply situated area. These examples are intended to underscore the pivotal importance of surgical biopsies in procuring comprehensive tissue samples essential for accurate diagnosis.Endoscopic biopsy is a medical procedure that allows doctors to examine the inside of the body using an endoscope, a flexible tube with a light and camera attached [
]. This procedure is commonly used to obtain tissue samples from the digestive tract or other internal organs for further examination. Figure 2 shows an endoscopic biopsy. For example, if a patient is experiencing unexplained digestive symptoms, such as persistent abdominal pain or unexplained weight loss, a gastroenterologist may perform an endoscopic biopsy to obtain tissue samples from the lining of the esophagus, stomach, or intestines. These samples can then be examined under a microscope to look for signs of inflammation, infection, or cancer.Similarly, in the case of suspected liver disease, an endoscopic biopsy can be used to obtain a small sample of liver tissue for analysis [
, ]. This can help doctors diagnose conditions such as cirrhosis, hepatitis, or liver cancer. Overall, endoscopic biopsy is a valuable tool in diagnosing and monitoring a wide range of medical conditions affecting the digestive system and other internal organs. The results of a biopsy are crucial in determining the presence and nature of any abnormal cells, which is vital for making an accurate diagnosis and developing an appropriate treatment plan for the patient.X-rays, CT scans, MRI scans, ultrasound, and PET scans are used to create detailed pictures of the inside of the body to identify tumors and determine their size and location [
, ]. Figure 3 displays various types of scans utilized for the diagnosis of cancer [ ].X-rays use electromagnetic radiation to create images of the inside of the body. They are commonly used to identify bone fractures, joint dislocations, and infections. For example, if someone has a suspected broken bone, an X-ray can show the location and severity of the fracture. CT scans, or computed tomography scans, use a series of X-ray images taken from different angles to create cross-sectional images of the body. This allows doctors to see inside the body in more detail than with a standard X-ray. CT scans are often used to identify tumors, internal bleeding, and other conditions. For instance, if a patient has a suspected brain tumor, a CT scan can provide detailed information about the size and location of the tumor [
, ].MRI scans, or magnetic resonance imaging scans, use a magnetic field and radio waves to create detailed images of the body's organs and tissues. MRI scans are particularly useful for imaging the brain, spinal cord, and joints. They can help identify tumors, injuries, and abnormalities in these areas [
, ]. For example, an MRI scan can show the size and location of a tumor in the brain. Ultrasound, also known as sonography, uses high-frequency sound waves to create images of the inside of the body. It is commonly used to examine the abdomen, pelvis, and blood vessels. Ultrasound is often used to identify tumors, monitor fetal development during pregnancy, and guide certain medical procedures [ , ]. For instance, an ultrasound can help identify the size and location of a tumor in the liver.PET scans, or positron emission tomography scans, involve the use of a radioactive substance called a tracer to show how organs and tissues are functioning. PET scans are often used to detect cancer, assess the spread of cancer, and evaluate the effectiveness of cancer treatment [
- ]. For example, a PET scan can show the metabolic activity of a tumor and help determine the best course of treatment. These imaging techniques play a crucial role in diagnosing and monitoring various medical conditions, including tumors. Each technique has its own strengths and limitations, and doctors may choose a specific imaging method based on the patient's symptoms and medical history.Blood tests are an important tool in the detection and monitoring of cancer, as shown in Figure 4. Tumor markers are substances that are produced by cancer cells or by the body in response to cancer. These markers can be detected in the blood and may indicate the presence of cancer [
, ]. Examples of tumor markers include prostate-specific antigen (PSA) for prostate cancer, CA-125 for ovarian cancer, and CEA for colorectal cancer [ - ].In addition to tumor markers, blood tests can also detect genetic mutations that are associated with certain types of cancer. For example, the BRCA1 and BRCA2 genes are known to be associated with an increased risk of breast and ovarian cancer. Testing for these genetic mutations can help identify individuals who may be at higher risk for developing these types of cancer. Furthermore, advancements in technology have led to the development of liquid biopsy tests, which can detect circulating tumor DNA in the blood. These tests can provide valuable information about the genetic makeup of a tumor, which can help guide treatment decisions and monitor the response to therapy.
Overall, blood tests play a crucial role in the early detection, diagnosis, and management of cancer and on-going research continues to expand the potential applications of blood-based biomarkers in oncology.
Staging plays a vital role in the diagnosis and treatment of cancer. Take breast cancer, for instance, to illustrate its importance. The staging system commonly used for breast cancer is the TNM system, which stands for tumor, node, and metastasis, as shown in Table 1 [
]. The T category (tumor size) describes the size of the primary tumor. For instance, a T1 tumor is generally smaller than a T2 or T3 tumor. The N category (lymph node involvement) indicates whether the cancer has spread to nearby lymph nodes. If cancer cells are found in the lymph nodes, it may indicate a more advanced stage of the disease. Finally, the M category (metastasis) reveals whether the cancer has spread to distant parts of the body.Let's say a patient is diagnosed with breast cancer, and after thorough testing, it's determined that the tumor is relatively small (T1), there is no evidence of cancer in the lymph nodes (N0), and there are no signs of distant metastasis (M0). This would be classified as an early stage of breast cancer, which generally has a better prognosis and may be treated differently than a more advanced stage [
]. By using the TNM system and other staging methods, healthcare professionals can better understand the extent of the cancer within the body, predict the prognosis, and tailor treatment plans to the specific stage of the disease. This personalized approach can significantly impact the patient's outcome and quality of life (Table 2).Histopathological analysis is a crucial aspect of cancer diagnosis and prognosis [
]. When a tissue sample is obtained from a patient, it is processed and examined under a microscope by a pathologist. The pathologist looks for specific characteristics in the cancer cells, such as their size, shape, and how they are arranged. These features help determine the type of cancer and its grade, which refers to how abnormal the cells look and how quickly they are likely to grow and spread. For example, in breast cancer, histopathological analysis can reveal whether the cancer is ductal or lobular, which can have implications for treatment decisions [ - ]. In addition, the grade of the cancer, such as whether it is low, intermediate, or high grade, can provide important information about its aggressiveness and potential response to treatment.Clinical examples of histopathological analysis include the examination of biopsy samples from patients with suspected or diagnosed cancer, as shown in Figure 5. By studying the cellular characteristics of the tumor, pathologists can provide valuable information to oncologists, helping guide treatment decisions and predict the likely course of the disease. Finally, histopathological analysis plays a critical role in understanding the nature of cancer at a cellular level, which is essential for making informed decisions about treatment and predicting patient outcomes.
Molecular testing in cancer involves examining the genetic characteristics of cancer cells to identify specific mutations or biomarkers [
]. These tests can provide valuable information for guiding treatment decisions and predicting prognosis. For example, in the case of non-small cell lung cancer, molecular testing can identify mutations in genes such as EGFR, ALK, ROS1, and BRAF. These mutations can help determine whether a patient is a candidate for targeted therapies, such as EGFR inhibitors or ALK inhibitors, which can be more effective than traditional chemotherapy in these specific cases.Another example is the use of molecular testing to guide treatment decisions in breast cancer. Testing for the presence of the HER2 gene amplification can identify patients who may benefit from targeted therapies like trastuzumab (Herceptin). Furthermore, in colorectal cancer, molecular testing for specific biomarkers, such as KRAS and NRAS mutations, can help predict the response to certain targeted therapies, such as anti-EGFR antibodies. Figure 6 shows molecular testing of lung cancer. By analyzing the genetic makeup of cancer cells, healthcare providers can tailor treatment plans to individual patients, leading to more personalized and effective care [
- ]. This approach is at the forefront of precision medicine, where treatments are customized based on the unique genetic characteristics of each patient's cancer. These traditional methods have been the cornerstone of cancer diagnosis and prognosis prediction for many years, and they continue to play a crucial role in the comprehensive assessment of cancer patients.Cancer diagnosis and prognosis prediction have made significant strides in recent years, but there are still limitations that need to be addressed. One of the primary limitations is the reliance on traditional histopathological analysis, which can be subjective and prone to inter-observer variability. This can lead to inconsistencies in diagnosis and prognosis prediction. Additionally, current techniques often rely on static snapshots of the tumor, which may not fully capture the dynamic nature of cancer progression. This can lead to inaccuracies in predicting how a tumor will respond to treatment and how it will evolve over time.
To address these limitations, more advanced techniques are being developed. For example, liquid biopsy, which involves analyzing tumor-derived materials such as circulating tumor cells and cell-free DNA in the blood, shows promise in providing real-time information about the tumor's genetic makeup and evolution. This can potentially offer a more comprehensive and dynamic view of the tumor compared to traditional biopsy methods. Another advanced technique is the use of AI and ML algorithms to analyze complex data sets, such as genomic and imaging data, to identify patterns and make more accurate predictions about a patient's prognosis. These techniques have the potential to revolutionize cancer diagnosis and prognosis prediction by providing more personalized and precise assessments.
Real-time examples of these advanced techniques in action can be found in on-going clinical trials and research studies. For instance, researchers are using liquid biopsy to monitor treatment response and detect the emergence of treatment-resistant mutations in real time. Similarly, ML algorithms are being applied to large-scale genomic and clinical data to develop predictive models that can help clinicians make more informed decisions about treatment strategies [
- ]. In conclusion, while there are limitations in current cancer diagnosis and prognosis prediction techniques, the development of more advanced approaches, such as liquid biopsy and ML, holds great promise for improving the accuracy and timeliness of cancer assessments. These advancements have the potential to transform the way we diagnose and predict the course of cancer, ultimately leading to more effective and personalized treatment strategies.FL is a ML approach that allows multiple institutions to collaborate on model training without sharing sensitive data [
]. This is particularly useful in fields like healthcare, where patient privacy is of utmost importance. The process includes the following steps :Initialization: A central server initializes the global model.
Distribution: The global model is then sent to each institution's local server.
Training: Each local server trains the model on its own dataset, using the data that is stored locally and never shared. This ensures that sensitive patient information remains within the institution.
Aggregation: After training, the updated models from all the local servers are sent back to the central server, which aggregates the changes to create an improved global model.
Iteration: This process of distribution, training, and aggregation is repeated for multiple iterations, allowing the global model to learn from the collective knowledge of all institutions without exposing individual patient data.
Here's a simple diagram to illustrate the process: Initially, the global model is sent to local servers at each institution, where it trains on local data, as shown in Figure 7. The updated models are then sent back to the central server for aggregation, resulting in an improved global model. This process is repeated iteratively, allowing the global model to learn from the collective knowledge of all institutions while preserving data privacy [
].In FL, instead of sending raw data to a central server, the model is sent to the data. Each institution trains the model on its local data, and only the updates to the model, rather than the raw data, are sent back to the central server. The central server then aggregates these updates to improve the global model. This way, the raw data never leaves the local institution, ensuring privacy and security.
Consider a healthcare scenario where several hospitals collaborate to develop a model aimed at predicting the progression of a specific disease. Each hospital has its own patient data, which is sensitive and private. With FL, these hospitals can collaborate on training the model without sharing individual patient records. Instead, they only share the model updates, allowing the global model to improve without compromising patient privacy. This approach not only protects sensitive data but also allows for a more diverse and representative dataset, as each institution's data may reflect different demographics or characteristics. FL thus enables institutions to work together to create more robust and accurate models while respecting data privacy and security.
Finally, FL is a powerful approach that enables collaboration on model training across multiple institutions without compromising the privacy of sensitive data [
]. It has significant implications for fields like healthcare, where data privacy is paramount, and it allows for the development of more robust and accurate models through collaborative efforts.Conventional ML approaches rely on centralized datasets collected from multiple institutions. Although conventional methods often yield high accuracy , they pose data privacy risks and require extensive data-sharing agreements. Table 3 provides a comparative analysis of conventional machine learning and Federated Learning.
Federated Learning’s decentralized nature significantly enhances data privacy but introduces communication overhead and requires robust synchronization mechanisms. However, conventional ML models still outperform FL in environments with limited computational resources due to FL’s dependency on distributed devices.
FL in cancer diagnosis is indeed a promising approach that addresses the challenge of data privacy while enabling collaborative model training. Let's delve into some real-time examples to illustrate its potential. In image analysis, consider a scenario where multiple hospitals possess diverse sets of medical images for cancer diagnosis. Instead of centralizing these images, FL allows the hospitals to collaboratively train a ML model without sharing the raw data [
, ]. Each hospital can locally train the model on its own data and then share only the model updates with a central server. The server aggregates these updates to improve the model without ever accessing the original images, thus preserving patient privacy.Similarly, in genomics, different research institutions may have genomic data relevant to cancer research. FL enables these institutions to jointly train models using their respective genomic datasets without sharing the actual genetic information. This collaborative approach allows for the development of more robust and generalizable models while respecting the privacy and security of sensitive patient data. By leveraging FL, institutions can benefit from the collective knowledge embedded in their decentralized datasets while upholding data privacy and security. This approach presents significant opportunities for advancing cancer diagnosis and treatment through the power of collaborative ML.
FL is indeed a promising approach for preserving data privacy while enabling the development of robust ML models. In the context of pathology, multiple healthcare institutions can collaborate to train a model on diverse datasets without sharing the actual data. For example, in cancer diagnosis, different hospitals can use FL to collaboratively train a model to identify cancerous cells in pathology images without sharing sensitive patient information. Similarly, in radiology, FL can be employed to train models for tasks such as detecting abnormalities in medical images [
]. Different hospitals or imaging centers can contribute their data to collectively train a model without the need to centralize the data, thus maintaining patient privacy and data security.One real-time example of FL in action is the use of this approach to develop AI models for diagnosing diabetic retinopathy [
]. Multiple healthcare providers can collaborate to train a model to detect signs of diabetic retinopathy in retinal images, ensuring that patient data remains within the respective institutions while benefiting from the collective knowledge gained through FL. Overall, FL holds great potential for advancing diagnostic modalities such as pathology and radiology by allowing the development of robust and generalizable models while upholding data privacy and security.FL is a ML approach that enables model training across multiple decentralized devices or servers holding local data samples, without exchanging them. This approach is particularly promising in the context of healthcare, where patient data privacy is of utmost importance. In the case of cancer prognosis prediction, FL can be used to aggregate insights from diverse patient populations while keeping sensitive data localized. For example, let's consider a scenario where different hospitals or research institutions each hold data on cancer patients [
]. Instead of pooling all this data into a central repository, which raises privacy concerns, FL allows the model to be trained across these distributed datasets.Here's how it works: Each institution trains a local model on its own data, learning from the unique characteristics of its patient population. The local models then send their insights (in the form of model updates) to a central server, where these updates are aggregated to improve the global model. This process iterates, with the global model becoming increasingly accurate without ever directly accessing individual patient data, as shown in Figure 8 [
]. By leveraging FL, healthcare providers can develop more accurate prognosis prediction models that reflect the diversity of cancer patients. This can lead to more personalized treatment plans tailored to the specific characteristics of each patient's cancer, potentially improving outcomes and reducing unnecessary treatments.For instance, imagine a global model trained using FL that accurately predicts the prognosis of breast cancer patients [
]. This model could take into account variations in tumor characteristics, genetic profiles, and treatment responses across different populations, leading to more personalized and effective treatment recommendations for individual patients. Furthermore, FL can also enable continuous learning and adaptation of the model as new data becomes available, ensuring that the prognosis prediction remains up-to-date and reflective of the latest insights from diverse patient populations.Overall, FL holds great promise in improving prognosis prediction in cancer patients by harnessing the collective knowledge within decentralized datasets while respecting patient privacy and data security. This approach has the potential to revolutionize cancer treatment by enabling more personalized and effective care strategies.
Implementing FL in the context of cancer diagnosis and prognosis prediction presents both challenges and opportunities. FL allows multiple institutions to collaborate on building a shared ML model without sharing sensitive patient data. However, several challenges need to be addressed for successful implementation.
One of the primary challenges in FL for cancer diagnosis is the heterogeneity of data across different healthcare institutions. Each institution may have different types of data, such as imaging data, genomic data, and clinical data, which can vary in format, quality, and quantity. This heterogeneity can make it difficult to train a unified model that performs well across all institutions.
To address this challenge, FL can be used to train models locally at each institution using their heterogeneous data. These local models can then be aggregated to create a global model that captures the diversity of data across institutions [
]. For example, different hospitals may have different types of imaging machines that produce data in different formats. FL models can be adapted to manage cross-institutional data heterogeneity and still make accurate predictions.Another challenge is the communication overhead associated with FL. In a FL setting, models are trained locally at each institution, and only model updates are shared with a central server for aggregation. However, the communication of these updates can be bandwidth-intensive, especially when dealing with large models and a large number of participating institutions.
To mitigate communication overhead, techniques such as model compression and differential privacy can be employed. Model compression techniques reduce the size of model updates before transmission, while differential privacy techniques add noise to the updates to protect the privacy of individual patient data [
, ]. For instance, in the context of cancer prognosis prediction, model compression can be used to reduce the size of the updates related to large-scale genomic data, making communication more efficient.Model aggregation in FL involves combining the local model updates from different institutions to create a global model. However, this process can be challenging due to variations in data distributions and quality across institutions. Aggregating models from heterogeneous data sources without compromising performance is a non-trivial task [
].To address this challenge, advanced aggregation techniques such as weighted averaging, secure aggregation, and meta-learning can be employed. Weighted averaging assigns different weights to local models based on their performance or data quality, allowing the global model to adapt to the characteristics of each institution's data. Secure aggregation techniques ensure that model updates are aggregated in a privacy-preserving manner, protecting sensitive patient information. Meta-learning techniques can be used to learn how to best combine models from different institutions over time, improving the overall performance of the global model.
GDPR (General Data Protection Regulation): The GDPR enforces strict data protection measures for patient data within the European Union. It mandates that personal health data should not be transferred outside the EU unless adequate safeguards are in place. FL aligns with GDPR principles by minimizing data transfer and ensuring data remains decentralized. However, ensuring full compliance with GDPR and HIPAA remains a key challenge with the right to be forgotten, data minimization, and consent management in FL systems.
HIPAA (Health Insurance Portability and Accountability Act): HIPAA regulates the use and disclosure of protected health information (PHI) in the United States. FL can aid compliance by reducing the risk of data breaches since patient data never leaves local institutions. However, ensuring adherence to access control policies, audit logging, and secure data storage remains a challenge in FL deployments.
To address privacy concerns, FL integrates a variety of privacy-preserving mechanisms, including :
Despite FL’s promising advantages, several challenges must be addressed before widespread adoption:
FL in cancer diagnosis and prognosis prediction indeed holds significant promise for the future of healthcare. This approach allows multiple institutions to collaborate on building robust ML models without sharing sensitive patient data. In the context of cancer diagnosis and prognosis prediction, FL enables the aggregation of diverse and large-scale datasets from different healthcare facilities, which can lead to more accurate and generalizable models. One of the key advantages of FL in this domain is the ability to train models on a wide range of patient populations, including those from different geographic locations and demographic backgrounds. This integration helps reduce dataset bias, promoting fairness in predictive outcomes in datasets from individual institutions and improve the overall performance and fairness of the predictive models.
Furthermore, FL can address privacy concerns associated with sharing sensitive medical data. By keeping the data localized and only sharing model updates, FL reduces the risk of data breaches and unauthorized access to patient information, thus maintaining patient privacy and confidentiality. However, there are also challenges associated with FL in healthcare, including the need for standardized data formats, interoperability of healthcare systems, and ensuring the security and integrity of the FL infrastructure. Additionally, issues concerning data quality, distribution imbalance, and inter-institutional variability across different institutions need to be carefully addressed to ensure the reliability and generalizability of the predictive models. Finally, while FL in cancer diagnosis and prognosis prediction offers great potential for advancing healthcare, it requires careful consideration of technical, ethical, and regulatory aspects to fully realize its benefits. As the field continues to evolve, addressing these challenges will be crucial in harnessing the power of FL for improving patient outcomes in cancer care.
Future directions include integrating multimodal data into FL models. By combining imaging, genomics, clinical, and other types of data from various sources, researchers can create more comprehensive models for cancer diagnosis and prognosis prediction. Furthermore, the impact on clinical practice could be substantial. FL models could enable more accurate and personalized cancer diagnoses, leading to earlier detection and intervention. This could ultimately improve patient outcomes and reduce healthcare costs.
Real-time examples of FL in cancer diagnosis and prognosis prediction might include a scenario where multiple hospitals collaborate to train a federated model for breast cancer detection using mammography images. Each hospital contributes its data to the model without sharing it externally, and the resulting model is then used to improve the accuracy of breast cancer screening across all participating institutions. Finally, FL demonstrates substantial potential to shape the future of precision cancer care , with potential research directions focusing on data heterogeneity and multimodal integration. The impact on clinical practice could lead to more accurate and personalized cancer care, ultimately benefiting patients and healthcare systems.
FL for cancer diagnosis and prognosis prediction demonstrates significant potential. Key findings indicate that this approach allows multiple institutions to collaborate on building robust ML models without sharing sensitive patient data. This is crucial for maintaining patient privacy and data security. The findings of this review suggest that the potential for more accurate and generalizable cancer diagnosis and prognosis prediction models, as well as the ability to leverage diverse datasets from different healthcare institutions. This could lead to improved patient outcomes and more personalized treatment plans.
Future research directions may include further refining FL algorithms to improve model performance, addressing challenges related to data heterogeneity and bias, and exploring the integration of FL into clinical practice. Additionally, efforts to establish standardized protocols for FL in healthcare and to ensure regulatory compliance will be important for widespread adoption.
Sheller MJ, Edwards B, Reina GA, Martin J, Pati S, Kotrotsou A, Milchenko M, Xu W, Marcus D, Colen RR, Bakas S. Federated learning in medicine: facilitating multi-institutional collaborations without sharing patient data. Sci Rep. 2020 Jul 28;10(1):12598. doi: 10.1038/s41598-020-69250-1. PMID: 32724046; PMCID: PMC7387485.
Maurya Y, Chandrahasan P. Federated learning for colorectal cancer prediction. In: 2022 IEEE 3rd Global Conference for Advancement in Technology (GCAT); IEEE. 2022;1-5.
Tan YN, Tinh VP, Lam PD, Nam NH, Khoa TA. A transfer learning approach to breast cancer classification in a federated learning framework. IEEE Access. 2023;11:27462-74.
Dasaradharami Reddy K, Gadekallu TR. A Comprehensive Survey on Federated Learning Techniques for Healthcare Informatics. Comput Intell Neurosci. 2023 Mar 1;2023:8393990. doi: 10.1155/2023/8393990. PMID: 36909974; PMCID: PMC9995203.
Kandati DR, Anusha S. Security and privacy in federated learning: A survey. Trends Comput Sci Inf Technol. 2023;8(2):029-37.
Srivastava G, Kandati DR, Yenduri G, Hegde P, Gadekallu TR, Maddikunta PKR, Bhattacharya S. Federated learning enabled edge computing security for Internet of Medical Things: Concepts, challenges and open issues. In: Security and Risk Analysis for Intelligent Edge Computing; Cham: Springer International Publishing; 2023. 67-89.
Chemmalar GS, Ramalingam M, Yenduri G, Raj Deepti G, Dasari Bhulakshmi, Dasaradharami KR, Supriya Y, Reddy Thippa G, Singh Rajkumar R, Jhaveri HR. The amalgamation of federated learning and explainable artificial intelligence for the Internet of Medical Things: A review. Recent Adv Comput Sci Commun. 2024;17:e121223224367. https://dx.doi.org/10.2174/0126662558266152231128060222
Rahman A, Hossain MS, Muhammad G, Kundu D, Debnath T, Rahman M, Khan MSI, Tiwari P, Band SS. Federated learning-based AI approaches in smart healthcare: concepts, taxonomies, challenges and open issues. Cluster Comput. 2022 Aug 17:1-41. doi: 10.1007/s10586-022-03658-4. Epub ahead of print. PMID: 35996680; PMCID: PMC9385101.
Gu X, Sabrina F, Fan Z, Sohail S. A Review of Privacy Enhancement Methods for Federated Learning in Healthcare Systems. Int J Environ Res Public Health. 2023 Aug 7;20(15):6539. doi: 10.3390/ijerph20156539. PMID: 37569079; PMCID: PMC10418741.
Subramanian M, Rajasekar V, VE S, Shanmugavadivel K, Nandhini PS. Effectiveness of decentralized federated learning algorithms in healthcare: A case study on cancer classification. Electronics. 2022;11(24):4117.
Kandati DR, Gadekallu TR. Genetic clustered federated learning for COVID-19 detection. Electronics. 2022;11(17):2714.
Gupta M, Kumar M, Gupta Y. A blockchain-empowered federated learning-based framework for data privacy in lung disease detection system. Comput Human Behav. 2024;158:108302.
Yadav G, Annappa B, Sachin DN. Abdominal multi-organ segmentation using federated learning. In: 2024 IEEE Region 10 Symposium (TENSYMP). 2024;1-7.
Brisimi TS, Chen R, Mela T, Olshevsky A, Paschalidis IC, Shi W. Federated learning of predictive models from federated Electronic Health Records. Int J Med Inform. 2018 Apr;112:59-67. doi: 10.1016/j.ijmedinf.2018.01.007. Epub 2018 Jan 12. PMID: 29500022; PMCID: PMC5836813.
Li T, Sanjabi M, Beirami A, Smith V. Fair resource allocation in federated learning. arXiv preprint arXiv:1905.10497. 2019.
Choudhury O, Park Y, Salonidis T, Gkoulalas-Divanis A, Sylla I, Das AK. Predicting Adverse Drug Reactions on Distributed Health Data using Federated Learning. AMIA Annu Symp Proc. 2020 Mar 4;2019:313-322. PMID: 32308824; PMCID: PMC7153050.
Rønn Hansen C, Price G, Field M, Sarup N, Zukauskaite R, Johansen J, Eriksen JG, Aly F, McPartlin A, Holloway L, Thwaites D, Brink C. Larynx cancer survival model developed through open-source federated learning. Radiother Oncol. 2022 Nov;176:179-186. doi: 10.1016/j.radonc.2022.09.023. Epub 2022 Oct 5. PMID: 36208652.
Sheller MJ, Edwards B, Reina GA, Martin J, Pati S, Kotrotsou A, Milchenko M, Xu W, Marcus D, Colen RR, Bakas S. Federated learning in medicine: facilitating multi-institutional collaborations without sharing patient data. Sci Rep. 2020 Jul 28;10(1):12598. doi: 10.1038/s41598-020-69250-1. PMID: 32724046; PMCID: PMC7387485.
Xu J, Glicksberg BS, Su C, Walker P, Bian J, Wang F. Federated Learning for Healthcare Informatics. J Healthc Inform Res. 2021;5(1):1-19. doi: 10.1007/s41666-020-00082-4. Epub 2020 Nov 12. PMID: 33204939; PMCID: PMC7659898.
Corti C, Cobanaj M, Dee EC, Criscitiello C, Tolaney SM, Celi LA, Curigliano G. Artificial intelligence in cancer research and precision medicine: Applications, limitations and priorities to drive transformation in the delivery of equitable and unbiased care. Cancer Treat Rev. 2023 Jan;112:102498. doi: 10.1016/j.ctrv.2022.102498. Epub 2022 Dec 11. PMID: 36527795.
Bhinder B, Gilvary C, Madhukar NS, Elemento O. Artificial Intelligence in Cancer Research and Precision Medicine. Cancer Discov. 2021 Apr;11(4):900-915. doi: 10.1158/2159-8290.CD-21-0090. PMID: 33811123; PMCID: PMC8034385.
Pulumati A, Pulumati A, Dwarakanath BS, Verma A, Papineni RVL. Technological advancements in cancer diagnostics: Improvements and limitations. Cancer Rep (Hoboken). 2023 Feb;6(2):e1764. doi: 10.1002/cnr2.1764. Epub 2023 Jan 6. PMID: 36607830; PMCID: PMC9940009.
Senthil Kumar K, Miskovic V, Blasiak A, Sundar R, Pedrocchi ALG, Pearson AT, Prelaj A, Ho D. Artificial Intelligence in Clinical Oncology: From Data to Digital Pathology and Treatment. Am Soc Clin Oncol Educ Book. 2023 May;43:e390084. doi: 10.1200/EDBK_390084. PMID: 37235822.
Shreve JT, Khanani SA, Haddad TC. Artificial Intelligence in Oncology: Current Capabilities, Future Opportunities, and Ethical Considerations. Am Soc Clin Oncol Educ Book. 2022 Apr;42:1-10. doi: 10.1200/EDBK_350652. PMID: 35687826.
Mitra S, Dey P. Fine-needle aspiration and core biopsy in the diagnosis of breast lesions: A comparison and review of the literature. Cytojournal. 2016 Aug 31;13:18. doi: 10.4103/1742-6413.189637. PMID: 27651820; PMCID: PMC5019018.
McAllister CM, Stepanian JD. The impact of minimally invasive surgical techniques on early range of motion after primary total knee arthroplasty. J Arthroplasty. 2008 Jan;23(1):10-8. doi: 10.1016/j.arth.2007.01.011. PMID: 18165022.
Darzi SA, Munz Y. The impact of minimally invasive surgical techniques. Annu Rev Med. 2004;55:223-37. doi: 10.1146/annurev.med.55.091902.105248. PMID: 14746519.
Iannessi A, Bertrand AS, Peyrottes I, Thyss A, Machiavello JC, Liberatore M. One-bloc percutaneous large biopsy of soft-tissue tumours: feasibility study and possible indications. Clin Radiol. 2019 Aug;74(8):649.e11-649.e17. doi: 10.1016/j.crad.2019.05.004. Epub 2019 Jun 6. PMID: 31178068.
Boese A, Wex C, Croner R, Liehr UB, Wendler JJ, Weigt J, Walles T, Vorwerk U, Lohmann CH, Friebe M, Illanes A. Endoscopic Imaging Technology Today. Diagnostics (Basel). 2022 May 18;12(5):1262. doi: 10.3390/diagnostics12051262. PMID: 35626417; PMCID: PMC9140648.
Johnson KD, Laoveeravat P, Yee EU, Perisetti A, Thandassery RB, Tharian B. Endoscopic ultrasound guided liver biopsy: Recent evidence. World J Gastrointest Endosc. 2020 Mar 16;12(3):83-97. doi: 10.4253/wjge.v12.i3.83. PMID: 32218888; PMCID: PMC7085945.
Pandey N, Hoilat GJ, John S. Liver biopsy. 2017.
Frangioni JV. New technologies for human cancer imaging. J Clin Oncol. 2008 Aug 20;26(24):4012-21. doi: 10.1200/JCO.2007.14.3065. PMID: 18711192; PMCID: PMC2654310.
García-Figueiras R, Baleato-González S, Padhani AR, Luna-Alcalá A, Vallejo-Casas JA, Sala E, Vilanova JC, Koh DM, Herranz-Carnero M, Vargas HA. How clinical imaging can assess cancer biology. Insights Imaging. 2019 Mar 4;10(1):28. doi: 10.1186/s13244-019-0703-0. PMID: 30830470; PMCID: PMC6399375.
Perez Giraldo GS, Singer L, Cao T, Jamshidi P, Dixit K, Kontzialis M, Castellani R, Pytel P, Anadani N, Bevan CJ, Grebenciucova E, Balabanov R, Cohen BA, Graham EL. Differential Diagnosis of Tumor-like Brain Lesions. Neurol Clin Pract. 2023 Oct;13(5):e200182. doi: 10.1212/CPJ.0000000000200182. Epub 2023 Aug 30. PMID: 37664132; PMCID: PMC10468256.
Huisman TA. Tumor-like lesions of the brain. Cancer Imaging. 2009 Oct 2;9 Spec No A(Special issue A):S10-3. doi: 10.1102/1470-7330.2009.9003. PMID: 19965288; PMCID: PMC2797474.
Dean Deyle G. The role of MRI in musculoskeletal practice: a clinical perspective. J Man Manip Ther. 2011 Aug;19(3):152-61. doi: 10.1179/2042618611Y.0000000009. PMID: 22851878; PMCID: PMC3143009.
Shah LM, Salzman KL. Imaging of spinal metastatic disease. Int J Surg Oncol. 2011;2011:769753. doi: 10.1155/2011/769753. Epub 2011 Nov 3. PMID: 22312523; PMCID: PMC3263660.
Silverstein J, Post AL, Chien AJ, Olin R, Tsai KK, Ngo Z, Van Loon K. Multidisciplinary Management of Cancer During Pregnancy. JCO Oncol Pract. 2020 Sep;16(9):545-557. doi: 10.1200/OP.20.00077. PMID: 32910882.
Sorouri K, Loren AW, Amant F, Partridge AH. Patient-Centered Care in the Management of Cancer During Pregnancy. Am Soc Clin Oncol Educ Book. 2023 May;43:e100037. doi: 10.1200/EDBK_100037. PMID: 37220323.
Griffeth LK. Use of PET/CT scanning in cancer patients: technical and practical considerations. Proc (Bayl Univ Med Cent). 2005 Oct;18(4):321-30. doi: 10.1080/08998280.2005.11928089. PMID: 16252023; PMCID: PMC1255942.
Mankoff DA, Katz SI. PET imaging for assessing tumor response to therapy. J Surg Oncol. 2018 Aug;118(2):362-373. doi: 10.1002/jso.25114. Epub 2018 Jun 24. PMID: 29938396.
Zhu A, Lee D, Shim H. Metabolic positron emission tomography imaging in cancer detection and therapy response. Semin Oncol. 2011 Feb;38(1):55-69. doi: 10.1053/j.seminoncol.2010.11.012. PMID: 21362516; PMCID: PMC3075495.
Li R, Ravizzini GC, Gorin MA, Maurer T, Eiber M, Cooperberg MR, Alemozzaffar M, Tollefson MK, Delacroix SE, Chapin BF. The use of PET/CT in prostate cancer. Prostate Cancer Prostatic Dis. 2018 Apr;21(1):4-21. doi: 10.1038/s41391-017-0007-8. Epub 2017 Dec 11. PMID: 29230009.
Deliu I, Cristina M, Dumitru G. Utility of tumor markers as a diagnostic tool. Curr Trends Nat Sci. 2018;7(14):272-5.
Nagpal M, Singh S, Singh P, Chauhan P, Zaidi MA. Tumor markers: A diagnostic tool. Natl J Maxillofac Surg. 2016 Jan-Jun;7(1):17-20. doi: 10.4103/0975-5950.196135. PMID: 28163473; PMCID: PMC5242068.
Desai S, Guddati AK. Carcinoembryonic Antigen, Carbohydrate Antigen 19-9, Cancer Antigen 125, Prostate-Specific Antigen and Other Cancer Markers: A Primer on Commonly Used Cancer Markers. World J Oncol. 2023 Feb;14(1):4-14. doi: 10.14740/wjon1425. Epub 2023 Feb 26. PMID: 36895994; PMCID: PMC9990734.
Basu A, Seth S, Chauhan AK, Bansal N, Arora K, Mahaur A. Comparative study of tumor markers in patients with colorectal carcinoma before and after chemotherapy. Ann Transl Med. 2016 Feb;4(4):71. doi: 10.3978/j.issn.2305-5839.2016.02.02. PMID: 27004218; PMCID: PMC4779780.
Sharma S. Tumor markers in clinical practice: General principles and guidelines. Indian J Med Paediatr Oncol. 2009 Jan;30(1):1-8. doi: 10.4103/0971-5851.56328. PMID: 20668599; PMCID: PMC2902207.
Rosen RD, Sapra A. TNM classification. In: StatPearls [Internet]. Treasure Island (FL): StatPearls Publishing. 2022.
Sawaki M, Shien T, Iwata H. TNM classification of malignant tumors (Breast Cancer Study Group). Jpn J Clin Oncol. 2019 Mar 1;49(3):228-231. doi: 10.1093/jjco/hyy182. PMID: 30541035.
Chen RJ, Lu MY, Wang J, Williamson DFK, Rodig SJ, Lindeman NI, Mahmood F. Pathomic Fusion: An Integrated Framework for Fusing Histopathology and Genomic Features for Cancer Diagnosis and Prognosis. IEEE Trans Med Imaging. 2022 Apr;41(4):757-770. doi: 10.1109/TMI.2020.3021387. Epub 2022 Apr 1. PMID: 32881682; PMCID: PMC10339462.
Tseng LJ, Matsuyama A, MacDonald-Dickinson V. Histology: The gold standard for diagnosis? Can Vet J. 2023 Apr;64(4):389-391. PMID: 37008634; PMCID: PMC10031787.
Zeiser FA, da Costa CA, Roehe AV, da Rosa Righi R, Marques NMC. Breast cancer intelligent analysis of histopathological data: A systematic review. Appl Soft Comput. 2021;113:107886.
Rashmi R, Prasad K, Udupa CBK. Breast histopathological image analysis using image processing techniques for diagnostic puposes: A methodological review. J Med Syst. 2021 Dec 3;46(1):7. doi: 10.1007/s10916-021-01786-9. PMID: 34860316; PMCID: PMC8642363.
Dabeer S, Khan MM, Islam S. Cancer diagnosis in histopathological image: CNN based approach. Informatics Med Unlocked. 2019;16:100231.
Sarhadi VK, Armengol G. Molecular Biomarkers in Cancer. Biomolecules. 2022 Jul 23;12(8):1021. doi: 10.3390/biom12081021. PMID: 35892331; PMCID: PMC9331210.
Sokolenko AP, Imyanitov EN. Molecular Diagnostics in Clinical Oncology. Front Mol Biosci. 2018 Aug 27;5:76. doi: 10.3389/fmolb.2018.00076. PMID: 30211169; PMCID: PMC6119963.
Malone ER, Oliva M, Sabatini PJB, Stockley TL, Siu LL. Molecular profiling for precision cancer therapies. Genome Med. 2020 Jan 14;12(1):8. doi: 10.1186/s13073-019-0703-1. PMID: 31937368; PMCID: PMC6961404.
Fares J, Fares MY, Khachfe HH, Salhab HA, Fares Y. Molecular principles of metastasis: a hallmark of cancer revisited. Signal Transduct Target Ther. 2020 Mar 12;5(1):28. doi: 10.1038/s41392-020-0134-x. PMID: 32296047; PMCID: PMC7067809.
Litton JK, Burstein HJ, Turner NC. Molecular Testing in Breast Cancer. Am Soc Clin Oncol Educ Book. 2019 Jan;39:e1-e7. doi: 10.1200/EDBK_237715. Epub 2019 May 17. PMID: 31099622.
Tang S, Yuan K, Chen L. Molecular biomarkers, network biomarkers, and dynamic network biomarkers for diagnosis and prediction of rare diseases. Fundam Res. 2022 Aug 9;2(6):894-902. doi: 10.1016/j.fmre.2022.07.011. PMID: 38933388; PMCID: PMC11197705.
El-Deiry WS, Goldberg RM, Lenz HJ, Shields AF, Gibney GT, Tan AR, Brown J, Eisenberg B, Heath EI, Phuphanich S, Kim E, Brenner AJ, Marshall JL. The current state of molecular testing in the treatment of patients with solid tumors, 2019. CA Cancer J Clin. 2019 Jul;69(4):305-343. doi: 10.3322/caac.21560. Epub 2019 May 22. PMID: 31116423; PMCID: PMC6767457.
Johnson KB, Wei WQ, Weeraratne D, Frisse ME, Misulis K, Rhee K, Zhao J, Snowdon JL. Precision Medicine, AI, and the Future of Personalized Health Care. Clin Transl Sci. 2021 Jan;14(1):86-93. doi: 10.1111/cts.12884. Epub 2020 Oct 12. PMID: 32961010; PMCID: PMC7877825.
Quazi S. Artificial intelligence and machine learning in precision and genomic medicine. Med Oncol. 2022 Jun 15;39(8):120. doi: 10.1007/s12032-022-01711-1. PMID: 35704152; PMCID: PMC9198206.
Habehh H, Gohel S. Machine Learning in Healthcare. Curr Genomics. 2021 Dec 16;22(4):291-300. doi: 10.2174/1389202922666210705124359. PMID: 35273459; PMCID: PMC8822225.
Qi P, Chiaro D, Guzzo A, Ianni M, Fortino G, Piccialli F. Model aggregation techniques in federated learning: A comprehensive survey. Future Gener Comput Syst. 2023.
Kandati DR, Gadekallu TR. Federated learning approach for early detection of chest lesion caused by COVID-19 infection using particle swarm optimization. Electronics. 2023;12(3):710.
Truong N, Sun K, Wang S, Guitton F, Guo Y. Privacy preservation in federated learning: An insightful survey from the GDPR perspective. Comput Secur. 2021;110:102402.
Sandhu SS, Gorji HT, Tavakolian P, Tavakolian K, Akhbardeh A. Medical Imaging Applications of Federated Learning. Diagnostics (Basel). 2023 Oct 6;13(19):3140. doi: 10.3390/diagnostics13193140. PMID: 37835883; PMCID: PMC10572559.
Sohan MF, Basalamah A. A systematic review on federated learning in medical image analysis. IEEE Access. 2023.
Nazir S, Kaleem M. Federated Learning for Medical Image Analysis with Deep Neural Networks. Diagnostics (Basel). 2023 Apr 24;13(9):1532. doi: 10.3390/diagnostics13091532. PMID: 37174925; PMCID: PMC10177193.
Padhy SK, Takkar B, Chawla R, Kumar A. Artificial intelligence in diabetic retinopathy: A natural step to the future. Indian J Ophthalmol. 2019 Jul;67(7):1004-1009. doi: 10.4103/ijo.IJO_1989_18. PMID: 31238395; PMCID: PMC6611318.
Walskaar I, Tran MC, Catak FO. A practical implementation of medical privacy-preserving federated learning using multi-key homomorphic encryption and Flower framework. Cryptography. 2023;7(4):48.
Rakhshan P. Breast cancer detection based on CNN and federated learning using embedded devices. 2023.
Carter SM, Rogers W, Win KT, Frazer H, Richards B, Houssami N. The ethical, legal and social implications of using artificial intelligence systems in breast cancer care. Breast. 2020 Feb;49:25-32. doi: 10.1016/j.breast.2019.10.001. Epub 2019 Oct 11. PMID: 31677530; PMCID: PMC7375671.
Zhu X, Wang J, Chen W, Sato K. Model compression and privacy preserving framework for federated learning. Future Gener Comput Syst. 2023;140:376-89.
Li Y, Li Y, Xu H, Ren S. An adaptive communication-efficient federated learning to resist gradient-based reconstruction attacks. Secur Commun Netw. 2021;2021:1-16.
Dasaradharami Reddy K, Srivastava G, Zhu Y, Supriya Y, Yenduri G, Victor N, et al. Federated Learning Using the Particle Swarm Optimization Model for the Early Detection of COVID-19. In: International Conference on Neural Information Processing; Nov 2023; Singapore: Springer Nature Singapore. 2023;425-36.
Dasaradharami Reddy K, Anusha S, Ashalatha N. A Comprehensive Review of Federated Learning in Cancer Diagnosis and Prognosis Prediction. IgMin Res. April 07, 2025; 3(4): 142-154. IgMin ID: igmin294; DOI:10.61927/igmin294; Available at: igmin.link/p294
任何您分享以下链接的人都可以阅读此内容:
1Assistant Professor, Department of Computer Science and Engineering, NBKR Institute of Science and Technology, Vidyanagar, India
2Assistant Professor, Department of Computer Science and Engineering, Annamacharya Institute of Technology and Sciences, Tirupati, India
Address Correspondence:
Dasaradharami Reddy K, Assistant Professor, Department of Computer Science and Engineering, NBKR Institute of Science and Technology, Vidyanagar, India, Email: [email protected]
How to cite this article:
Dasaradharami Reddy K, Anusha S, Ashalatha N. A Comprehensive Review of Federated Learning in Cancer Diagnosis and Prognosis Prediction. IgMin Res. April 07, 2025; 3(4): 142-154. IgMin ID: igmin294; DOI:10.61927/igmin294; Available at: igmin.link/p294
Copyright: © 2025 Dasaradharami Reddy K, et al. This is an open access article distributed under the Creative Commons Attribution License, which permits unrestricted use, distribution, and reproduction in any medium, provided the original work is properly cited.
Sheller MJ, Edwards B, Reina GA, Martin J, Pati S, Kotrotsou A, Milchenko M, Xu W, Marcus D, Colen RR, Bakas S. Federated learning in medicine: facilitating multi-institutional collaborations without sharing patient data. Sci Rep. 2020 Jul 28;10(1):12598. doi: 10.1038/s41598-020-69250-1. PMID: 32724046; PMCID: PMC7387485.
Maurya Y, Chandrahasan P. Federated learning for colorectal cancer prediction. In: 2022 IEEE 3rd Global Conference for Advancement in Technology (GCAT); IEEE. 2022;1-5.
Tan YN, Tinh VP, Lam PD, Nam NH, Khoa TA. A transfer learning approach to breast cancer classification in a federated learning framework. IEEE Access. 2023;11:27462-74.
Dasaradharami Reddy K, Gadekallu TR. A Comprehensive Survey on Federated Learning Techniques for Healthcare Informatics. Comput Intell Neurosci. 2023 Mar 1;2023:8393990. doi: 10.1155/2023/8393990. PMID: 36909974; PMCID: PMC9995203.
Kandati DR, Anusha S. Security and privacy in federated learning: A survey. Trends Comput Sci Inf Technol. 2023;8(2):029-37.
Srivastava G, Kandati DR, Yenduri G, Hegde P, Gadekallu TR, Maddikunta PKR, Bhattacharya S. Federated learning enabled edge computing security for Internet of Medical Things: Concepts, challenges and open issues. In: Security and Risk Analysis for Intelligent Edge Computing; Cham: Springer International Publishing; 2023. 67-89.
Chemmalar GS, Ramalingam M, Yenduri G, Raj Deepti G, Dasari Bhulakshmi, Dasaradharami KR, Supriya Y, Reddy Thippa G, Singh Rajkumar R, Jhaveri HR. The amalgamation of federated learning and explainable artificial intelligence for the Internet of Medical Things: A review. Recent Adv Comput Sci Commun. 2024;17:e121223224367. https://dx.doi.org/10.2174/0126662558266152231128060222
Rahman A, Hossain MS, Muhammad G, Kundu D, Debnath T, Rahman M, Khan MSI, Tiwari P, Band SS. Federated learning-based AI approaches in smart healthcare: concepts, taxonomies, challenges and open issues. Cluster Comput. 2022 Aug 17:1-41. doi: 10.1007/s10586-022-03658-4. Epub ahead of print. PMID: 35996680; PMCID: PMC9385101.
Gu X, Sabrina F, Fan Z, Sohail S. A Review of Privacy Enhancement Methods for Federated Learning in Healthcare Systems. Int J Environ Res Public Health. 2023 Aug 7;20(15):6539. doi: 10.3390/ijerph20156539. PMID: 37569079; PMCID: PMC10418741.
Subramanian M, Rajasekar V, VE S, Shanmugavadivel K, Nandhini PS. Effectiveness of decentralized federated learning algorithms in healthcare: A case study on cancer classification. Electronics. 2022;11(24):4117.
Kandati DR, Gadekallu TR. Genetic clustered federated learning for COVID-19 detection. Electronics. 2022;11(17):2714.
Gupta M, Kumar M, Gupta Y. A blockchain-empowered federated learning-based framework for data privacy in lung disease detection system. Comput Human Behav. 2024;158:108302.
Yadav G, Annappa B, Sachin DN. Abdominal multi-organ segmentation using federated learning. In: 2024 IEEE Region 10 Symposium (TENSYMP). 2024;1-7.
Brisimi TS, Chen R, Mela T, Olshevsky A, Paschalidis IC, Shi W. Federated learning of predictive models from federated Electronic Health Records. Int J Med Inform. 2018 Apr;112:59-67. doi: 10.1016/j.ijmedinf.2018.01.007. Epub 2018 Jan 12. PMID: 29500022; PMCID: PMC5836813.
Li T, Sanjabi M, Beirami A, Smith V. Fair resource allocation in federated learning. arXiv preprint arXiv:1905.10497. 2019.
Choudhury O, Park Y, Salonidis T, Gkoulalas-Divanis A, Sylla I, Das AK. Predicting Adverse Drug Reactions on Distributed Health Data using Federated Learning. AMIA Annu Symp Proc. 2020 Mar 4;2019:313-322. PMID: 32308824; PMCID: PMC7153050.
Rønn Hansen C, Price G, Field M, Sarup N, Zukauskaite R, Johansen J, Eriksen JG, Aly F, McPartlin A, Holloway L, Thwaites D, Brink C. Larynx cancer survival model developed through open-source federated learning. Radiother Oncol. 2022 Nov;176:179-186. doi: 10.1016/j.radonc.2022.09.023. Epub 2022 Oct 5. PMID: 36208652.
Sheller MJ, Edwards B, Reina GA, Martin J, Pati S, Kotrotsou A, Milchenko M, Xu W, Marcus D, Colen RR, Bakas S. Federated learning in medicine: facilitating multi-institutional collaborations without sharing patient data. Sci Rep. 2020 Jul 28;10(1):12598. doi: 10.1038/s41598-020-69250-1. PMID: 32724046; PMCID: PMC7387485.
Xu J, Glicksberg BS, Su C, Walker P, Bian J, Wang F. Federated Learning for Healthcare Informatics. J Healthc Inform Res. 2021;5(1):1-19. doi: 10.1007/s41666-020-00082-4. Epub 2020 Nov 12. PMID: 33204939; PMCID: PMC7659898.
Corti C, Cobanaj M, Dee EC, Criscitiello C, Tolaney SM, Celi LA, Curigliano G. Artificial intelligence in cancer research and precision medicine: Applications, limitations and priorities to drive transformation in the delivery of equitable and unbiased care. Cancer Treat Rev. 2023 Jan;112:102498. doi: 10.1016/j.ctrv.2022.102498. Epub 2022 Dec 11. PMID: 36527795.
Bhinder B, Gilvary C, Madhukar NS, Elemento O. Artificial Intelligence in Cancer Research and Precision Medicine. Cancer Discov. 2021 Apr;11(4):900-915. doi: 10.1158/2159-8290.CD-21-0090. PMID: 33811123; PMCID: PMC8034385.
Pulumati A, Pulumati A, Dwarakanath BS, Verma A, Papineni RVL. Technological advancements in cancer diagnostics: Improvements and limitations. Cancer Rep (Hoboken). 2023 Feb;6(2):e1764. doi: 10.1002/cnr2.1764. Epub 2023 Jan 6. PMID: 36607830; PMCID: PMC9940009.
Senthil Kumar K, Miskovic V, Blasiak A, Sundar R, Pedrocchi ALG, Pearson AT, Prelaj A, Ho D. Artificial Intelligence in Clinical Oncology: From Data to Digital Pathology and Treatment. Am Soc Clin Oncol Educ Book. 2023 May;43:e390084. doi: 10.1200/EDBK_390084. PMID: 37235822.
Shreve JT, Khanani SA, Haddad TC. Artificial Intelligence in Oncology: Current Capabilities, Future Opportunities, and Ethical Considerations. Am Soc Clin Oncol Educ Book. 2022 Apr;42:1-10. doi: 10.1200/EDBK_350652. PMID: 35687826.
Mitra S, Dey P. Fine-needle aspiration and core biopsy in the diagnosis of breast lesions: A comparison and review of the literature. Cytojournal. 2016 Aug 31;13:18. doi: 10.4103/1742-6413.189637. PMID: 27651820; PMCID: PMC5019018.
McAllister CM, Stepanian JD. The impact of minimally invasive surgical techniques on early range of motion after primary total knee arthroplasty. J Arthroplasty. 2008 Jan;23(1):10-8. doi: 10.1016/j.arth.2007.01.011. PMID: 18165022.
Darzi SA, Munz Y. The impact of minimally invasive surgical techniques. Annu Rev Med. 2004;55:223-37. doi: 10.1146/annurev.med.55.091902.105248. PMID: 14746519.
Iannessi A, Bertrand AS, Peyrottes I, Thyss A, Machiavello JC, Liberatore M. One-bloc percutaneous large biopsy of soft-tissue tumours: feasibility study and possible indications. Clin Radiol. 2019 Aug;74(8):649.e11-649.e17. doi: 10.1016/j.crad.2019.05.004. Epub 2019 Jun 6. PMID: 31178068.
Boese A, Wex C, Croner R, Liehr UB, Wendler JJ, Weigt J, Walles T, Vorwerk U, Lohmann CH, Friebe M, Illanes A. Endoscopic Imaging Technology Today. Diagnostics (Basel). 2022 May 18;12(5):1262. doi: 10.3390/diagnostics12051262. PMID: 35626417; PMCID: PMC9140648.
Johnson KD, Laoveeravat P, Yee EU, Perisetti A, Thandassery RB, Tharian B. Endoscopic ultrasound guided liver biopsy: Recent evidence. World J Gastrointest Endosc. 2020 Mar 16;12(3):83-97. doi: 10.4253/wjge.v12.i3.83. PMID: 32218888; PMCID: PMC7085945.
Pandey N, Hoilat GJ, John S. Liver biopsy. 2017.
Frangioni JV. New technologies for human cancer imaging. J Clin Oncol. 2008 Aug 20;26(24):4012-21. doi: 10.1200/JCO.2007.14.3065. PMID: 18711192; PMCID: PMC2654310.
García-Figueiras R, Baleato-González S, Padhani AR, Luna-Alcalá A, Vallejo-Casas JA, Sala E, Vilanova JC, Koh DM, Herranz-Carnero M, Vargas HA. How clinical imaging can assess cancer biology. Insights Imaging. 2019 Mar 4;10(1):28. doi: 10.1186/s13244-019-0703-0. PMID: 30830470; PMCID: PMC6399375.
Perez Giraldo GS, Singer L, Cao T, Jamshidi P, Dixit K, Kontzialis M, Castellani R, Pytel P, Anadani N, Bevan CJ, Grebenciucova E, Balabanov R, Cohen BA, Graham EL. Differential Diagnosis of Tumor-like Brain Lesions. Neurol Clin Pract. 2023 Oct;13(5):e200182. doi: 10.1212/CPJ.0000000000200182. Epub 2023 Aug 30. PMID: 37664132; PMCID: PMC10468256.
Huisman TA. Tumor-like lesions of the brain. Cancer Imaging. 2009 Oct 2;9 Spec No A(Special issue A):S10-3. doi: 10.1102/1470-7330.2009.9003. PMID: 19965288; PMCID: PMC2797474.
Dean Deyle G. The role of MRI in musculoskeletal practice: a clinical perspective. J Man Manip Ther. 2011 Aug;19(3):152-61. doi: 10.1179/2042618611Y.0000000009. PMID: 22851878; PMCID: PMC3143009.
Shah LM, Salzman KL. Imaging of spinal metastatic disease. Int J Surg Oncol. 2011;2011:769753. doi: 10.1155/2011/769753. Epub 2011 Nov 3. PMID: 22312523; PMCID: PMC3263660.
Silverstein J, Post AL, Chien AJ, Olin R, Tsai KK, Ngo Z, Van Loon K. Multidisciplinary Management of Cancer During Pregnancy. JCO Oncol Pract. 2020 Sep;16(9):545-557. doi: 10.1200/OP.20.00077. PMID: 32910882.
Sorouri K, Loren AW, Amant F, Partridge AH. Patient-Centered Care in the Management of Cancer During Pregnancy. Am Soc Clin Oncol Educ Book. 2023 May;43:e100037. doi: 10.1200/EDBK_100037. PMID: 37220323.
Griffeth LK. Use of PET/CT scanning in cancer patients: technical and practical considerations. Proc (Bayl Univ Med Cent). 2005 Oct;18(4):321-30. doi: 10.1080/08998280.2005.11928089. PMID: 16252023; PMCID: PMC1255942.
Mankoff DA, Katz SI. PET imaging for assessing tumor response to therapy. J Surg Oncol. 2018 Aug;118(2):362-373. doi: 10.1002/jso.25114. Epub 2018 Jun 24. PMID: 29938396.
Zhu A, Lee D, Shim H. Metabolic positron emission tomography imaging in cancer detection and therapy response. Semin Oncol. 2011 Feb;38(1):55-69. doi: 10.1053/j.seminoncol.2010.11.012. PMID: 21362516; PMCID: PMC3075495.
Li R, Ravizzini GC, Gorin MA, Maurer T, Eiber M, Cooperberg MR, Alemozzaffar M, Tollefson MK, Delacroix SE, Chapin BF. The use of PET/CT in prostate cancer. Prostate Cancer Prostatic Dis. 2018 Apr;21(1):4-21. doi: 10.1038/s41391-017-0007-8. Epub 2017 Dec 11. PMID: 29230009.
Deliu I, Cristina M, Dumitru G. Utility of tumor markers as a diagnostic tool. Curr Trends Nat Sci. 2018;7(14):272-5.
Nagpal M, Singh S, Singh P, Chauhan P, Zaidi MA. Tumor markers: A diagnostic tool. Natl J Maxillofac Surg. 2016 Jan-Jun;7(1):17-20. doi: 10.4103/0975-5950.196135. PMID: 28163473; PMCID: PMC5242068.
Desai S, Guddati AK. Carcinoembryonic Antigen, Carbohydrate Antigen 19-9, Cancer Antigen 125, Prostate-Specific Antigen and Other Cancer Markers: A Primer on Commonly Used Cancer Markers. World J Oncol. 2023 Feb;14(1):4-14. doi: 10.14740/wjon1425. Epub 2023 Feb 26. PMID: 36895994; PMCID: PMC9990734.
Basu A, Seth S, Chauhan AK, Bansal N, Arora K, Mahaur A. Comparative study of tumor markers in patients with colorectal carcinoma before and after chemotherapy. Ann Transl Med. 2016 Feb;4(4):71. doi: 10.3978/j.issn.2305-5839.2016.02.02. PMID: 27004218; PMCID: PMC4779780.
Sharma S. Tumor markers in clinical practice: General principles and guidelines. Indian J Med Paediatr Oncol. 2009 Jan;30(1):1-8. doi: 10.4103/0971-5851.56328. PMID: 20668599; PMCID: PMC2902207.
Rosen RD, Sapra A. TNM classification. In: StatPearls [Internet]. Treasure Island (FL): StatPearls Publishing. 2022.
Sawaki M, Shien T, Iwata H. TNM classification of malignant tumors (Breast Cancer Study Group). Jpn J Clin Oncol. 2019 Mar 1;49(3):228-231. doi: 10.1093/jjco/hyy182. PMID: 30541035.
Chen RJ, Lu MY, Wang J, Williamson DFK, Rodig SJ, Lindeman NI, Mahmood F. Pathomic Fusion: An Integrated Framework for Fusing Histopathology and Genomic Features for Cancer Diagnosis and Prognosis. IEEE Trans Med Imaging. 2022 Apr;41(4):757-770. doi: 10.1109/TMI.2020.3021387. Epub 2022 Apr 1. PMID: 32881682; PMCID: PMC10339462.
Tseng LJ, Matsuyama A, MacDonald-Dickinson V. Histology: The gold standard for diagnosis? Can Vet J. 2023 Apr;64(4):389-391. PMID: 37008634; PMCID: PMC10031787.
Zeiser FA, da Costa CA, Roehe AV, da Rosa Righi R, Marques NMC. Breast cancer intelligent analysis of histopathological data: A systematic review. Appl Soft Comput. 2021;113:107886.
Rashmi R, Prasad K, Udupa CBK. Breast histopathological image analysis using image processing techniques for diagnostic puposes: A methodological review. J Med Syst. 2021 Dec 3;46(1):7. doi: 10.1007/s10916-021-01786-9. PMID: 34860316; PMCID: PMC8642363.
Dabeer S, Khan MM, Islam S. Cancer diagnosis in histopathological image: CNN based approach. Informatics Med Unlocked. 2019;16:100231.
Sarhadi VK, Armengol G. Molecular Biomarkers in Cancer. Biomolecules. 2022 Jul 23;12(8):1021. doi: 10.3390/biom12081021. PMID: 35892331; PMCID: PMC9331210.
Sokolenko AP, Imyanitov EN. Molecular Diagnostics in Clinical Oncology. Front Mol Biosci. 2018 Aug 27;5:76. doi: 10.3389/fmolb.2018.00076. PMID: 30211169; PMCID: PMC6119963.
Malone ER, Oliva M, Sabatini PJB, Stockley TL, Siu LL. Molecular profiling for precision cancer therapies. Genome Med. 2020 Jan 14;12(1):8. doi: 10.1186/s13073-019-0703-1. PMID: 31937368; PMCID: PMC6961404.
Fares J, Fares MY, Khachfe HH, Salhab HA, Fares Y. Molecular principles of metastasis: a hallmark of cancer revisited. Signal Transduct Target Ther. 2020 Mar 12;5(1):28. doi: 10.1038/s41392-020-0134-x. PMID: 32296047; PMCID: PMC7067809.
Litton JK, Burstein HJ, Turner NC. Molecular Testing in Breast Cancer. Am Soc Clin Oncol Educ Book. 2019 Jan;39:e1-e7. doi: 10.1200/EDBK_237715. Epub 2019 May 17. PMID: 31099622.
Tang S, Yuan K, Chen L. Molecular biomarkers, network biomarkers, and dynamic network biomarkers for diagnosis and prediction of rare diseases. Fundam Res. 2022 Aug 9;2(6):894-902. doi: 10.1016/j.fmre.2022.07.011. PMID: 38933388; PMCID: PMC11197705.
El-Deiry WS, Goldberg RM, Lenz HJ, Shields AF, Gibney GT, Tan AR, Brown J, Eisenberg B, Heath EI, Phuphanich S, Kim E, Brenner AJ, Marshall JL. The current state of molecular testing in the treatment of patients with solid tumors, 2019. CA Cancer J Clin. 2019 Jul;69(4):305-343. doi: 10.3322/caac.21560. Epub 2019 May 22. PMID: 31116423; PMCID: PMC6767457.
Johnson KB, Wei WQ, Weeraratne D, Frisse ME, Misulis K, Rhee K, Zhao J, Snowdon JL. Precision Medicine, AI, and the Future of Personalized Health Care. Clin Transl Sci. 2021 Jan;14(1):86-93. doi: 10.1111/cts.12884. Epub 2020 Oct 12. PMID: 32961010; PMCID: PMC7877825.
Quazi S. Artificial intelligence and machine learning in precision and genomic medicine. Med Oncol. 2022 Jun 15;39(8):120. doi: 10.1007/s12032-022-01711-1. PMID: 35704152; PMCID: PMC9198206.
Habehh H, Gohel S. Machine Learning in Healthcare. Curr Genomics. 2021 Dec 16;22(4):291-300. doi: 10.2174/1389202922666210705124359. PMID: 35273459; PMCID: PMC8822225.
Qi P, Chiaro D, Guzzo A, Ianni M, Fortino G, Piccialli F. Model aggregation techniques in federated learning: A comprehensive survey. Future Gener Comput Syst. 2023.
Kandati DR, Gadekallu TR. Federated learning approach for early detection of chest lesion caused by COVID-19 infection using particle swarm optimization. Electronics. 2023;12(3):710.
Truong N, Sun K, Wang S, Guitton F, Guo Y. Privacy preservation in federated learning: An insightful survey from the GDPR perspective. Comput Secur. 2021;110:102402.
Sandhu SS, Gorji HT, Tavakolian P, Tavakolian K, Akhbardeh A. Medical Imaging Applications of Federated Learning. Diagnostics (Basel). 2023 Oct 6;13(19):3140. doi: 10.3390/diagnostics13193140. PMID: 37835883; PMCID: PMC10572559.
Sohan MF, Basalamah A. A systematic review on federated learning in medical image analysis. IEEE Access. 2023.
Nazir S, Kaleem M. Federated Learning for Medical Image Analysis with Deep Neural Networks. Diagnostics (Basel). 2023 Apr 24;13(9):1532. doi: 10.3390/diagnostics13091532. PMID: 37174925; PMCID: PMC10177193.
Padhy SK, Takkar B, Chawla R, Kumar A. Artificial intelligence in diabetic retinopathy: A natural step to the future. Indian J Ophthalmol. 2019 Jul;67(7):1004-1009. doi: 10.4103/ijo.IJO_1989_18. PMID: 31238395; PMCID: PMC6611318.
Walskaar I, Tran MC, Catak FO. A practical implementation of medical privacy-preserving federated learning using multi-key homomorphic encryption and Flower framework. Cryptography. 2023;7(4):48.
Rakhshan P. Breast cancer detection based on CNN and federated learning using embedded devices. 2023.
Carter SM, Rogers W, Win KT, Frazer H, Richards B, Houssami N. The ethical, legal and social implications of using artificial intelligence systems in breast cancer care. Breast. 2020 Feb;49:25-32. doi: 10.1016/j.breast.2019.10.001. Epub 2019 Oct 11. PMID: 31677530; PMCID: PMC7375671.
Zhu X, Wang J, Chen W, Sato K. Model compression and privacy preserving framework for federated learning. Future Gener Comput Syst. 2023;140:376-89.
Li Y, Li Y, Xu H, Ren S. An adaptive communication-efficient federated learning to resist gradient-based reconstruction attacks. Secur Commun Netw. 2021;2021:1-16.
Dasaradharami Reddy K, Srivastava G, Zhu Y, Supriya Y, Yenduri G, Victor N, et al. Federated Learning Using the Particle Swarm Optimization Model for the Early Detection of COVID-19. In: International Conference on Neural Information Processing; Nov 2023; Singapore: Springer Nature Singapore. 2023;425-36.